Lectures
This course is running at Penn during the Fall of 2023. This course is structured around recorded video lectures that students are expected to watch on Mondays followed by discussion sessions on Wednesdays and Fridays. Most video lectures last between 40 and 60 minutes, although some are a little longer. Follow this link to access lecture recordings and materials.
If you haven’t already realized this, please be aware that learning from a video is tough. This is why we have the discussion sessions but I also want you to make the most of the recorded lectures. To that end, you will see that individual lectures have been separated into modules that last between 3 and 10 minutes and that modules are aggregated in a playlist. This is intended for you to look at them separately or all together. Most people find that watching videos in short chunks is best.
Whatever your watching preferences, be aware that it is very easy to watch a video and not get anything out of it. To help you with that, in addition to recordings, you will see that each lecture comes with a handout and a script. If you put together the handout and the script, that’s the lecture for you. My idea is that you will be able to download the handout and the script and have them by your side as you watch the lecture. I am a big believer in associating space and concepts. I am hoping that the printed handout and script help you with that
Interactive Meetings
Depending on the session for which you are registered, we meet on Wednesdays and Fridays at 8:30 am in the LRSM Auditorium or at 10:15 am in the active learning classroom on the 4th floor of Walnut 3401. These meetings are reserved for interactions. Come prepared to talk. Be ready to ask questions. Be ready to answer questions.
Please reserve 90 minutes of your time for these meetings. That is, reserve Wednesdays and Fridays from 8:30 am to 10 am or 10:15 am to 11:45 am Eastern Time, depending on your section. However, we will try to keep the discussion sessions to 60-70 minutes instead of 90.
On the same Monday when we start work on a set of lectures, we also start work on a set of questions associated with each lecture. You will be randomly assigned to groups of size 3 or 4 each, which will be announced at the beginning of the semester. Each group is required to prepare 1 to 3 slides with their response to their assigned question as well as a speech lasting about 5 minutes. Group to question mappings are announced before the beginning of each week. During the interactive sessions, you will share the slides and speech with the rest of the class, and the instructor will provide feedback and open the floor for discussion. Half of the questions will be discussed on Wednesdays, and the other half will be discussed on Fridays. You can prepare your response using whatever software you choose. If you are a latex person, you can download a Penn-branded template.
The responses you will give during the interactive session are zero-stakes. Do your best and don’t stress. You are free to remember that your instructor failed Programming 101 and Electronics 101 when he was in college.
Lab Assignments
On the Mondays of Weeks 2, 4, 7, 10, and 13, we will release Lab assignments. These assignments will be due on the last day (Fridays mostly, but can be on the day before the Fall Break) of Weeks 3, 6, 9, 12, and 15. This means you have two or three work weeks to complete each assignment. The grades for each lab will be announced on the Monday following the lab due date. You will then have two days (until the end of Wednesday) to submit corrections if some of your answers are incorrect. If you miss the original due date on Friday, you may make a late submission until the following Wednesday and receive partial credit, without a chance for resubmission. More details on the grading criteria can be found below.
You will work in groups of three. We will send information about pairings, and you will keep these pairings throughout the term. The labs are designed to be worked on your own. The purpose of having teams is to give you a sounding board. If you are stuck on a question, chances are your partners are not. If the three of you are stuck on a questions, it may be a good idea to submit a question to the discussion board for the TAs to answer.
The TAs are going to arrange two blocks of 90 minutes per week where you can meet with them. Take advantage of these meetings. You don’t know your TAs yet, but I do. I can vouch that they are knowledgeable and hard working. They are also good people and will help you out as much as you need.
When you complete the assignment, you will have to submit a report for the group by having one group member submitting the report to Canvas. The labs will contain very concrete instructions on how to write the reports. I am interpreting the lab reports under the language of the Paperwork Reduction Act. They are intended for you to complete in less than one hour after you finish your work. No need for florid language, showing work, or code submission. For each question we will ask for a short deliverable. The goal is simply to check that your answers are correct. If your answers are note correct, we will get back to you to offer you a chance for corrections. As long as you implement these corrections, you will get full marks for the assignment if your grade is greater than or equal to 14, and partial marks if your grade is below 14. The link for the Canvas page can be found here.
The labs are designed to illustrate points I will be making in lectures. For example, Lab 1 will be about understanding the importance of choosing appropriate parametrizations when we are we solve a problem using learning techniques. I am telling you this because labs also have a heavy development component. You will have to write some code that is not difficult but not easy either. I don’t want you to loose focus on the fact that the objective of the lab is not to get the code working. Rather, the focus of the lab is to understand why your code works.
The labs will use the Python programming language, the PyTorch library for machine learning, and the Alelab Graph Neural Network library. In Lab 3 we are going to explain how to use the Alelab GNN library. However, we will assume from the start that you are familiar with Python and that you have succeeded at installing Pytorch. You don’t need to be familiar with Pytorch. We will go over the use of this library in Labs 1 and 2. For those of you who are not familiar with Python, and need to install Python and Pytorch, please refer to this guide that we have put together.
Discussion Forum
We are running a discussion Forum on Ed Discussion. Please be engaged. Submit questions. Answer questions. Offer comments. Offer help
Teaching Assistants
Shervin Khalafi (shervink@seas.upenn.edu) is a second-year PhD student at the University of Pennsylvania advised by Prof. Ribeiro. Currently, he is working on the theory of graph neural networks and their optimization. More broadly, his research interests include Optimization, GNNs and Learning theory. He is originally from Tehran, Iran, where he received a BSc. in Electrical Engineering from Sharif University of Technology.
Frederic Vatnsdal (vatnsdal@seas.upenn.edu) is a second-year PhD student advised by Prof. Alejandro Ribeiro. The focus of his research is on the learning and deployment of GNN-based decentralized control policies for robot swarms. Prior to Penn, he worked at Nvidia on their autonomous vehicles team. He received his BASc. in Electrical Engineering from the University of Waterloo in Canada.
Beiming Li (beimingl@seas.upenn.edu) is a first-year PhD student advised by Prof. Alejandro Ribeiro and Prof. Vijay Kumar. The focus of his research is on designing decentralized multi-agent systems with graph neural network and reinforcement learning. He received his BASc. in Computer Engineering from the University of Michigan and Electrical Engineering from Shanghai Jiao Tong University.
Jiashu He (jiashuhe@seas.upenn.edu) is a Ph.D. student in CIS at the University of Pennsylvania. He is interested in solving optimization problems using machine learning, and boosting reasoning ability of AI systems/LLMs using human cognitive inspired approaches. He received a BSc. in Mathematics and Computer Science from University of Illinois, Urbana Champaign.
Office Hours
Shervin: Mondays 12-1pm, 3401 Walnut, 4th floor, B wing Lounge.
Fred: Thursdays 1-2pm, 3401 Walnut, 4th floor, B wing Lounge.
Beiming: Wednesdays 2-3pm, Online (https://upenn.zoom.us/j/6104810433).
Jiashu: Wednesdays 3-4 pm, 3401 Walnut, 4th floor, B wing Lounge.
Grading
There are 5 labs in this course, and each of them counts for 16 points. If all of the answers you submit are correct, you get 20 points for the lab. If some of your answers are incorrect, you get a chance to submit corrections. After submitting your corrections, we will grade your resubmission and give you a maximum of 18 points for the lab. In case you skip the main due date and make a late submission (by the end of the following Wednesday), we will grade your late submission and give you a maximum of 16 points. No resubmissions are allowed in this case.
Lab scores are further weighted by class attendance. The formula for the final score is as follows,
$$ \text{final score} = (\text{lab score}) \times \text{min} (1, \text{attendance} / 20)$$
In this formula “lab score” is the sum of all of the lab reports. Since there are a total of 25 lectures during the term, the class attendance factor implies that you can skip 5 lectures without repercussion. If you skip more than 5 lectures, the “final score” decreases in proportion to the number of classes missed.
This grading rubric is designed so that if you submit all of your assignments on time and also submit the corrections, you get an A- for the course. You will get an A for the course if at least 3 of your original reports are correct on the first submission. This is assuming that you attend (and actively participate!) in the meetings. Your grade could go downhill quite quickly if you skip lectures, submit your assignments late or do not submit the assignments at all. While hard to believe, this does happen every year to some students.
Some of you are not getting credit for this course. We are inspired by your willingness to learn, but please keep us inspired. We just ask that you take the class as seriously as students that are officially registered. That is, watch the videos, show up to the interactive sessions, and complete the assignments.
Course Instructor
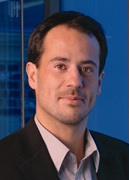
Alejandro Ribeiro (aribeiro@seas.upenn.edu) : I am an older version of the guy on the left and a Professor of Electrical and Systems Engineering (ESE) at the University of Pennsylvania (Penn). I was born in Montevideo, Uruguay where I studied and got an Electrical Engineering degree from the Universidad de la República in 1999. I worked as a systems engineer for a cellphone company until 2003 when I moved to the United States to study at the University of Minnesota (UoM) in Minneapolis. I received M. Sc. and Ph. D. degrees from the UoM in 2005 and 2008, respectively.
In case you need either, you can access my professional bio in this link and my academic cv in this link. These two documents are prepared by the serious person I am forced to impersonate every so often. To get a sense of my research and teaching activities please keep reading. For a more comprehensive discussion visit my lab’s website which has descriptions of my research vision and provides access to the sites for the courses I teach. I am hoping that you will find my lab’s blog to be illuminating and entertaining but if you are hard core, my list of papers is here.
I have had and continue to have the honor of working with remarkable doctoral students. Their papers have received a number of awards which I am happy to claim as my own. These awards are the 2020 Signal Processing Society young author best paper award, the 2014 O. Hugo Schuck best paper award and paper awards at the 2020 International Conference on Acoustics, Speech and Signal Processing, the 2019 European Signal Processing Conference, the 2017 Conference on Decision and Control, the 2016 Workshop on Statistical Signal Processing, the 2016 Sensor Array, and Multichannel Signal Processing Workshop, the 2015 Asilomar Conference on Signals Systems and computers, the 2013 American Control Conference, and the 2005 and 2006 International Conferences on Acoustics, Speech, and Signal Processing. I also have had and continue to have the honor of working with remarkable undergraduate students. I am very proud of their recognition in the form of the 2012 S. Reid Warren, Jr. Award presented by the undergraduate student body of Penn Engineering for outstanding teaching as well as the recognition of my colleagues with the Penn-wide 2017 Lindback award for distinguished teaching. I have also been selected as a Fulbright Scholar class of 2003 and a Penn Fellow class of 2015.